The use of machine learning (ML) has revolutionized many industries, and sports prediction is no exception. From football to basketball, tennis to cricket, machine learning algorithms have become an essential tool for analyzing data and making smarter predictions. Platforms like Playstat are at the forefront of this technological advancement, using machine learning to provide users with accurate, data-driven insights into upcoming games and events. In this article, we explore how machine learning is transforming sports prediction and how tools like Playstat can help sports fans, bettors, and analysts make more informed decisions.
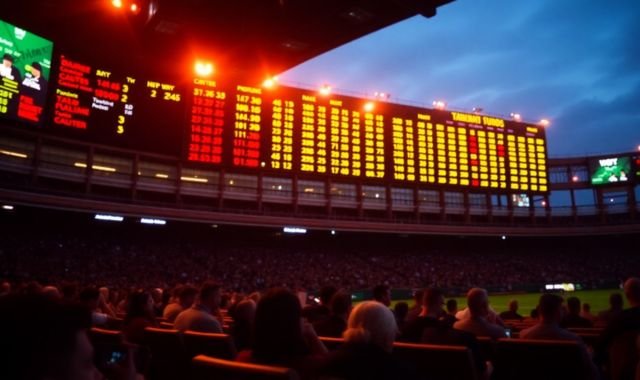
The Power of Machine Learning in Sports Prediction
Machine learning involves creating algorithms that allow computers to learn from data, identify patterns, and make predictions without human intervention. In sports, this means analyzing vast amounts of data — from player statistics and team performance metrics to historical match results and even weather conditions. The goal is to extract meaningful patterns that can inform future outcomes, allowing for smarter predictions.
At the core of machine learning’s application in sports prediction is its ability to process massive amounts of data far faster and more accurately than a human ever could. Traditionally, sports analysts would rely on intuition, basic statistics, and historical knowledge to predict match outcomes. Today, ML algorithms take these same data points and enhance them by identifying correlations that may not be immediately obvious, offering more reliable predictions.
How Playstat Leverages Machine Learning for Sports Predictions
Playstat is one of the leading platforms integrating machine learning into sports prediction. By employing sophisticated ML models, Playstat is able to generate accurate forecasts for a wide range of sports, including football, basketball, tennis, and more. The platform’s success lies in its ability to gather and process large datasets from a variety of sources and then apply machine learning techniques to predict the outcome of future events.
For instance, Playstat uses a variety of machine learning techniques like regression models, classification algorithms, and neural networks to forecast outcomes. These methods take into account not only player statistics and team performance but also external factors such as injuries, weather, and even public sentiment. As a result, Playstat can predict match results with a high level of accuracy, providing sports fans, analysts, and bettors with valuable insights.
If you want to learn more or discuss how Playstat can enhance your sports prediction strategy, feel free to reach out to the Playstat team at marketing@playstat.com.
Key Machine Learning Techniques Used in Sports Prediction
Machine learning is not a one-size-fits-all solution, and different algorithms serve different purposes in sports prediction. Let’s take a look at some of the key techniques used to make smarter sports predictions:
- Regression Analysis
Regression analysis is one of the simplest and most common ML techniques used in sports prediction. It involves identifying relationships between various variables and predicting a continuous outcome. In sports, regression models can predict variables like total points scored in a basketball game or the number of goals in a football match. These models are especially useful when analyzing team performance based on past results. - Classification Algorithms
While regression models predict continuous outcomes, classification algorithms are used for categorical predictions. For example, classification algorithms can be used to predict whether a team will win, lose, or draw based on a variety of input features. Decision trees, random forests, and support vector machines are some of the most common classification algorithms employed in sports prediction. - Neural Networks
Neural networks, a more complex and advanced form of machine learning, are highly effective in recognizing patterns and correlations in large datasets. These networks simulate the way human brains process information, making them particularly good at handling unstructured data like images or text. In sports prediction, neural networks can be used to process a variety of data points (team performance, player form, etc.) and provide more nuanced predictions. - Clustering and Unsupervised Learning
In sports prediction, unsupervised learning techniques like clustering are used to group similar teams, players, or events together. This allows machine learning models to make predictions based on the characteristics of similar historical instances. For example, clustering might identify certain teams that perform similarly under specific conditions (like home games or against certain opponents), which can inform future predictions. - Reinforcement Learning
Reinforcement learning is an emerging technique in sports prediction. This type of ML algorithm learns by trial and error, using feedback to adjust its predictions over time. In sports, this could be applied to real-time predictions, where the model continually improves its forecasts as it receives more data from ongoing games or seasons. This dynamic learning process helps the algorithm adapt to the ever-changing nature of sports.
Benefits of Machine Learning in Sports Prediction
Machine learning offers several distinct advantages when it comes to predicting sports outcomes:
- Accuracy: By analyzing large datasets and learning from historical patterns, machine learning algorithms can make predictions with a high degree of accuracy. This is particularly useful in sports, where minute details often determine the outcome of games.
- Real-Time Analysis: Machine learning models can analyze real-time data, including live match statistics, player injuries, and other variables, to adjust predictions during games. This makes it ideal for dynamic sports environments.
- Data-Driven Decisions: Machine learning removes much of the guesswork and bias from predictions. Instead of relying on subjective opinions or instincts, ML models use objective data to generate outcomes, making predictions more reliable.
- Scalability: As more data becomes available, machine learning models improve over time. This means that the more you use a platform like Playstat, the more refined and accurate its predictions become.
Why Playstat is a Game Changer for Sports Prediction
Playstat stands out in the crowded field of sports prediction tools due to its integration of machine learning. By using advanced algorithms to process vast amounts of data, Playstat offers highly accurate, real-time predictions across various sports. Whether you’re a casual fan looking to predict match outcomes for fun, or a serious bettor trying to gain an edge, Playstat’s machine learning-powered predictions can help you make smarter, data-driven decisions.
In addition to its powerful prediction algorithms, Playstat provides a user-friendly interface that simplifies the process of analyzing complex data. Even those with minimal experience in sports prediction can leverage Playstat’s insights, while more advanced users can dive deeper into the statistical models to refine their predictions further.
To get started with Playstat and see how machine learning can elevate your sports predictions, visit the platform and explore its various features. For any inquiries or assistance, feel free to reach out via email at marketing@playstat.com.
Conclusion
Machine learning is undeniably changing the way we approach sports prediction. By utilizing data-driven models, platforms like Playstat provide more accurate and timely insights, giving users an edge in predicting sports outcomes. The ability of machine learning algorithms to analyze vast amounts of data and uncover hidden patterns has made predictions more reliable than ever before. Whether you’re an avid sports fan or a seasoned bettor, embracing machine learning-powered platforms like Playstat can help you make smarter, more informed decisions in the world of sports prediction.